The GAN River-I data set is designed to provide a stern test for machine learning and geostatistical tools that wish to recreate the complex geometries of realistic facies distributions in subsurface reservoirs. It provides more complex, non-stationary facies distributions than previous open data sets, some of which have modelled channels but do not include the number and complex association of facies types of this data set.
GAN River-I is a dataset of 2D layers of 3D facies models produced from a process-based simulator of a meandering fluvial system. The data set contains 25 simulated 3D cubes, converted into three datasets consisting of 16,000 2D models/images, each representing the increasing complexity of the modelled facies. The number of facies decreases between the three data sets, with nine facies, seven facies and three facies, respectively. The facies reduction is carried out by amalgamating similar facies in terms of their likely permeability to represent flowing units in a subsurface reservoir. The data is therefore provided to allow users to increase the model complexity in a manageable and comparable way between groups using the data.
GAN River-I covers a range of low NTG meandering patterns with varied avulsion rates. Each dataset comprises an ensemble of meandering models representing various plausible patterns and, therefore, can be used as a geologically plausible benchmark for testing generative models’ performance. We provide three data file formats, including image, Ndarray and GSLIB, to adapt to different researchers’ preferences.
Reference: Chao Sun, Vasily Demyanov, Daniel Arnold (2022) GAN River-I: A process-based low NTG meandering reservoir model dataset for machine learning studies, Data in Brief, Elsevier. https://doi.org/10.1016/j.dib.2022.108785.
Download data from GitHub repository
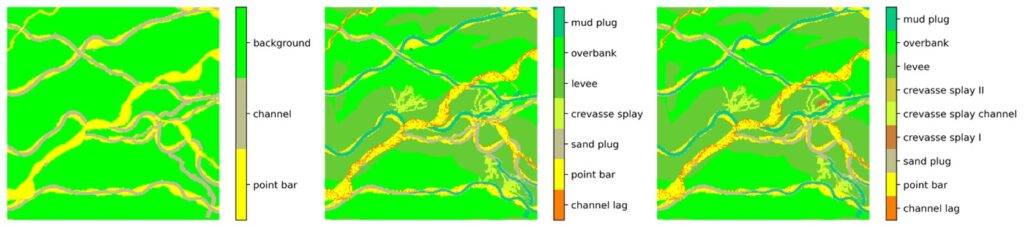