Challenges and Solutions in Stochastic Reservoir Modelling
By Vasily Demyanov , Dan Arnold, EAGE 2018, 260 p.

This book aims to bridge across different fields — geostatistics, machine learning, and Bayesian statistics — to demonstrate the common grounds in solving challenging problems of uncertainty quantification, geological realism, and data integration in reservoir prediction. It presents an overview of key concepts and some of the basic and more advanced algorithms for reservoir modelling and uncertainty quantification. This book includes several practical examples to reinforce the learning outcomes. A hand-on self-guided tutorial on decision making under uncertainty provides a practical way to apply integrated thinking to a real field dataset. The book was published for the EET 12 of the EAGE Education Tour series – Making the case for geostatistics and machine learning for reservoir modelling, First Break interview, Feb 2018
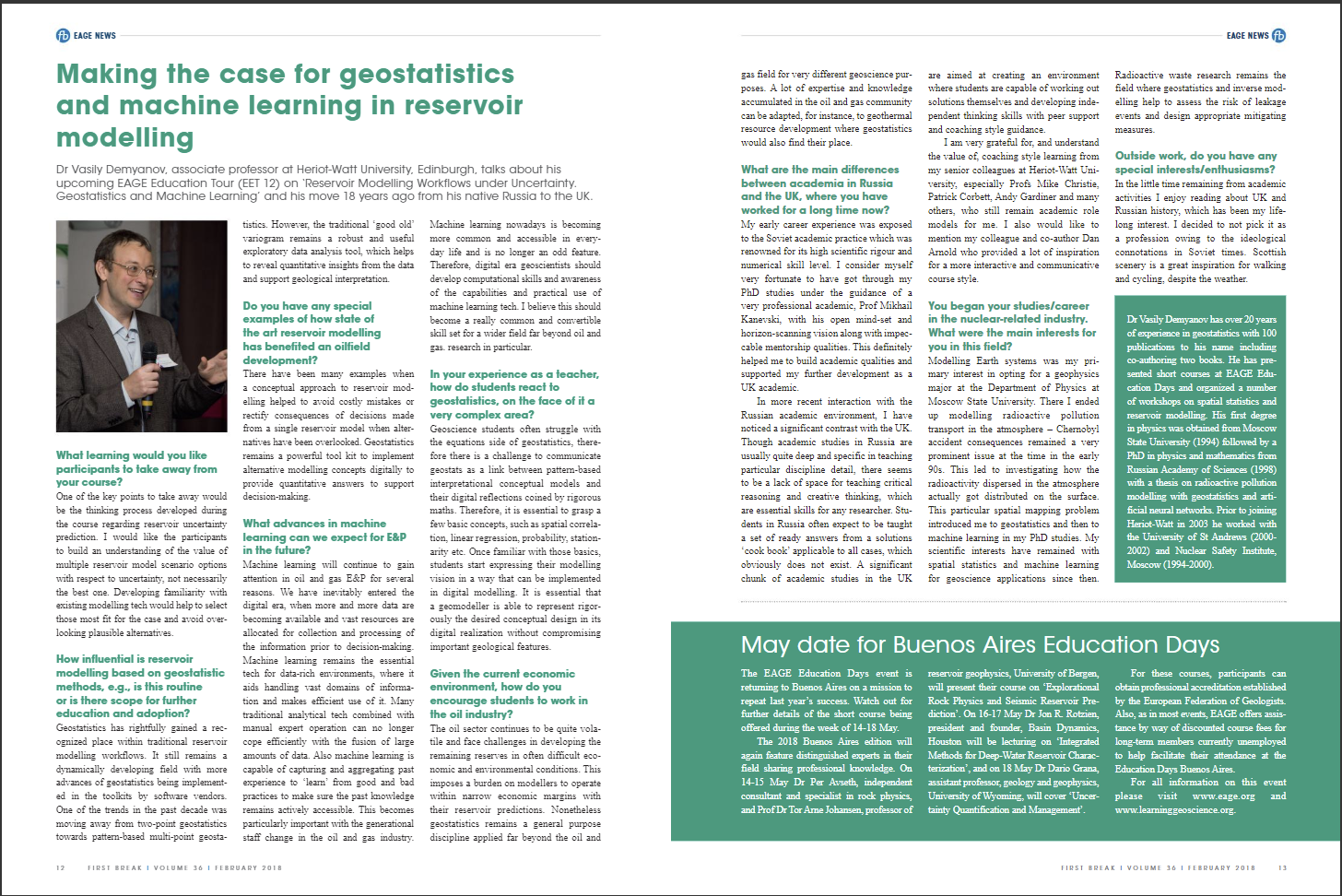
Table of contents
Preface 7
Content 9
1 INTRODUCTION 11
1.1 Introduction 13
1.2 Uncertainty and geostatistical modelling 14
1.3 The modelling workflow 17
1.4 Book structure 21
2 BASIC ASSUMPTIONS OF GEOSTATISTICS 23
2.1 Spatial continuity 25
2.2 Modelling approaches 26
2.3 Data support 28
2.4 Building a spatial model from data 30
2.5 Stochastic modelling nature and statistical assumptions 31
2.6 Characteristics of a spatial random process 34
3 DISCOVERY AND MODELLING OF SPATIAL CORRELATION USING VARIOGRAPHY 43
3.1 Spatial continuity and correlation 45
3.2 Spatial correlation described by variography 47
3.3 Variogram modelling 60
3.4 Summary 73
4 GEOSTATISTICAL ESTIMATION AND STOCHASTIC SIMULATION 75
4.1 Kriging 78
4.2 Stochastic simulations 99
4.3 Summary: geostatistical predictors and stochastic simulations 127
5 LEARNING-BASED MODELS FOR RESERVOIR DESCRIPTION 129
5.1 Learning from data — a concept for modelling 131
5.2 Learning algorithms 133
5.3 Modelling approaches and a model choice 134
5.4 Model complexity in a reservoir description — a simple example 140
5.5 Support vector models 142
5.6 Semi-supervised models for reservoir description 144
5.7 Feature selection with multiple kernel learning 147
5.8 Summary 15512
Content
6 UNCERTAINTY QUANTIFICATION OF RESERVOIR PREDICTION 157
6.1 Introduction to uncertainty quantification 159
6.2 Bayes’ theorem and history matching 163
6.3 Building the uncertainty model 168
6.4 Geological parameterisation and history matching 169
6.5 Geological priors in history matching and uncertainty quantification 174
6.6 Interpretation, model setup, and parameterisation 180
6.7 Application of uncertainty quantification workflow to a fracture reservoir model example 185
6.8 Summary 195
Exercises 197
Answers and recommendations to the exercises 246
BIBLIOGRAPHY 253
Index 259
Geostatistics: Theory and Practice (in Russian)
V. Demyanov, E. Savelieva, Nauka 2010, 329 p. (available for download)
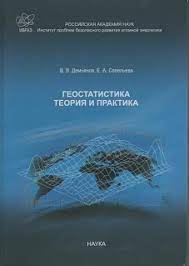
Simplicity, Complexity and Modelling
Mike Christie (Editor), Andrew Cliffe (Editor), Philip Dawid (Editor), Stephen S. Senn (Editor), Wiley, 2011, 220 pages
Several points of disagreement exist between different modelling traditions as to whether complex models are always better than simpler models, as to how to combine results from different models and how to propagate model uncertainty into forecasts. This book represents the result of collaboration between scientists from many disciplines to show how these conflicts can be resolved.
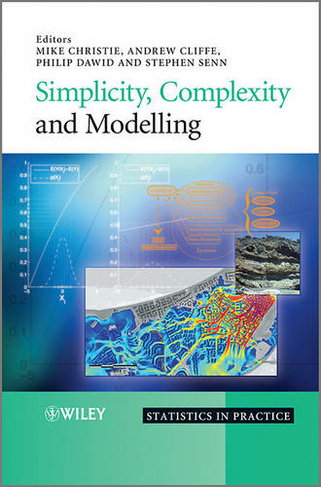
Key features:
- Introduces important concepts in modelling, outlining different traditions in the use of simple and complex modelling in statistics.
- Provides numerous case studies on complex modelling, such as climate change, flood risk and new drug development.
- Concentrates on varying models, including flood risk analysis models, the petrol industry forecasts and summarizes the evolution of water distribution systems.
- Written by experienced statisticians and engineers in order to facilitate communication between modellers in different disciplines.
- Provides a glossary giving terms commonly used in different modelling traditions.
This book provides a much-needed reference guide to approaching statistical modelling. Scientists involved with modelling complex systems in areas such as climate change, flood prediction and prevention, financial market modelling and systems engineering will benefit from this book. It will also be a useful source of modelling case histories.