patterns in geological & reservoir data
Geological and reservoir data feature patterns that reflect natural processes and manmade activity in complex systems. A challenge is to reveal these patterns given the combination of natural processes can be hugely complex and subject to uncertainty due to the unknown aspects of the physics.
Machine learning is design to learn patterns from data. It finds a number of applications in geological and porous media that reveal insights of natural system behavior and help to gain better understanding and build better models to make better decisions.
- Discover patterns in seismic
- Discover patterns in outcrop images
- Interpretational uncertainty in depositional scenario discovery from wireline log facies classification
- Uncertainty in facies predictions
- Surface lithology mapping with radiometry
- Big data analytics for pattern discovery in large reservoir basin property data bases
Discover patterns in seismic
Automated detection of geological structures from seismic – from 3D seismic cube to turbidite fan detection. Multiple seismic interpretation scenarios are detected from real seismic (Falkland basin) to describe the range of structural uncertainty. Automated machine learning based interpretation is comparable to the one done manually by BGS experts and reproduces realistic geological structures.
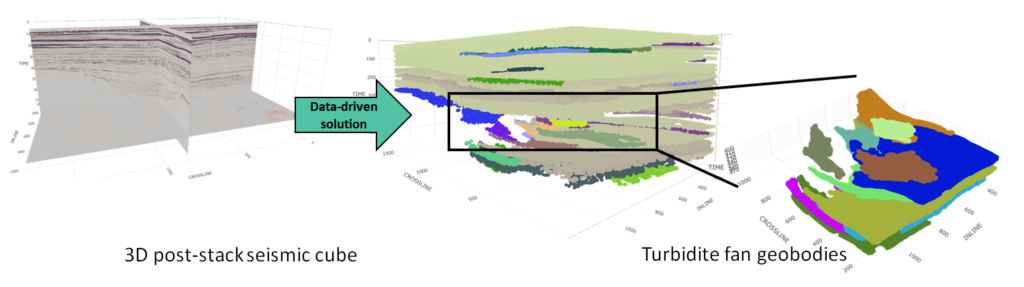
See more details in “Turbidite Fan Interpretation in 3D Seismic Data by Point Cloud Segmentation Using Machine Learning“, by Q. Corlay, V. Demyanov, D. McCarthy and D. Arnold, EAGE 2020 Annual Conference & Exhibition Online, Dec 2020.
Discover patterns in outcrop images
Use geological interpretations in training for classification sedimentary structures from outcrop images. Outcrop sedimentary structure classification is improved with using sketched interpretations along with the outcrops for training.
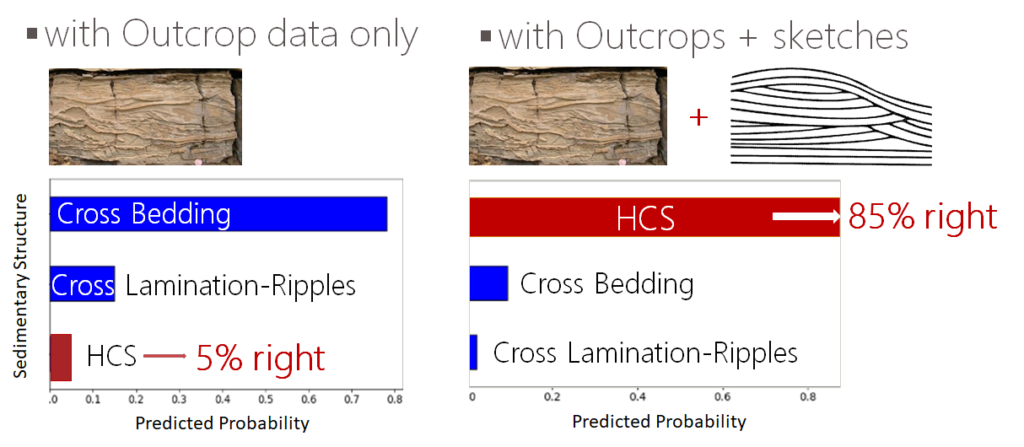
See more detains in “The Importance of Blending Different Data Types to Train Machine Learning Classifiers for Sedimentary Structure Detection” by A. Nathanail, V. Demyanov, D. Arnold, A. Gardiner, to be presented and EAGE 2021.
Depositional scenario discovery from wireline logs
Machine learning wireline log classification enables differentiation between alternative depositional scenarios – turbidite distal deposits and hybrid event beds (HEB).
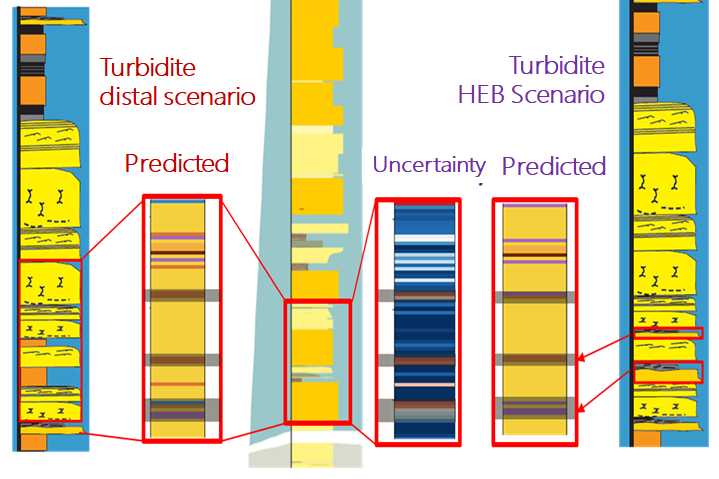
Falkland Basin study by Halotel, J., Demyanov, V. & Gardiner, A., British Sedimentological Research Group meeting 2018.
Uncertainty in facies predictions
Machine learning classification of facies from wireline logs tend to provide a single prediction with no much respect for uncertainty. This does not quite reflect the uncertain nature of facies classification based on wireline and core interpretation. This work demonstrates how uncertainty can be associated with facies classification with machine learning algorithm. A Random forest classifier was applied to a benchmark facies classification data set from the Panoma field, see Hall, M., & Hall, B. (2017). Distributed collaborative prediction: Results of the machine learning contest. The Leading Edge, 36(3), 267-269.
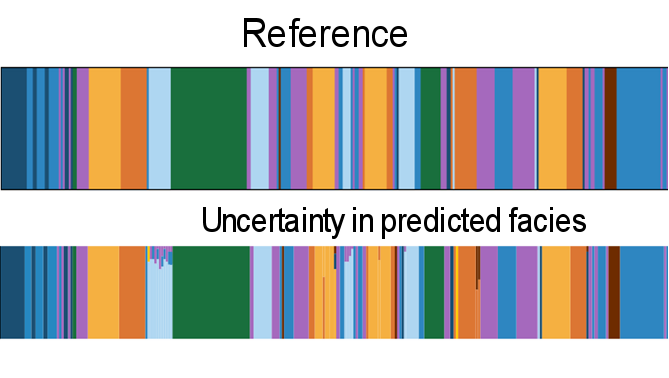
Halotel, J., Demyanov, V. & Gardiner, A., 16 Nov 2019, In: Mathematical Geosciences, 52, pages5–29 (2020)
Surface lithology mapping with radiometry
More accurate and detailed mapping of near surface lithology is obtained based on integration of radiometric data and other relevant geo features into unsupervised classification with Self-organising Maps. Tellus data study was performed in collaboration with Jennifer McKinley of Queen’s University Belfast and Mark Cooper with GSNI.
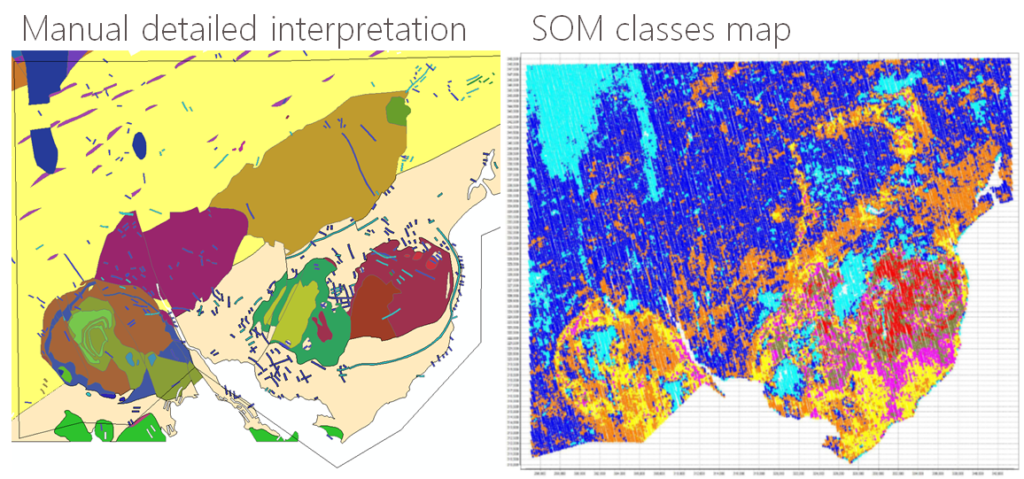
Smillie, Z., Demyanov, V., McKinley, J. & Cooper, M., 7 Jul 2023, In: Journal of the Geological Society. 180, 4, jgs2022-136.
Big data analytics for pattern discovery in large reservoir basin property data bases
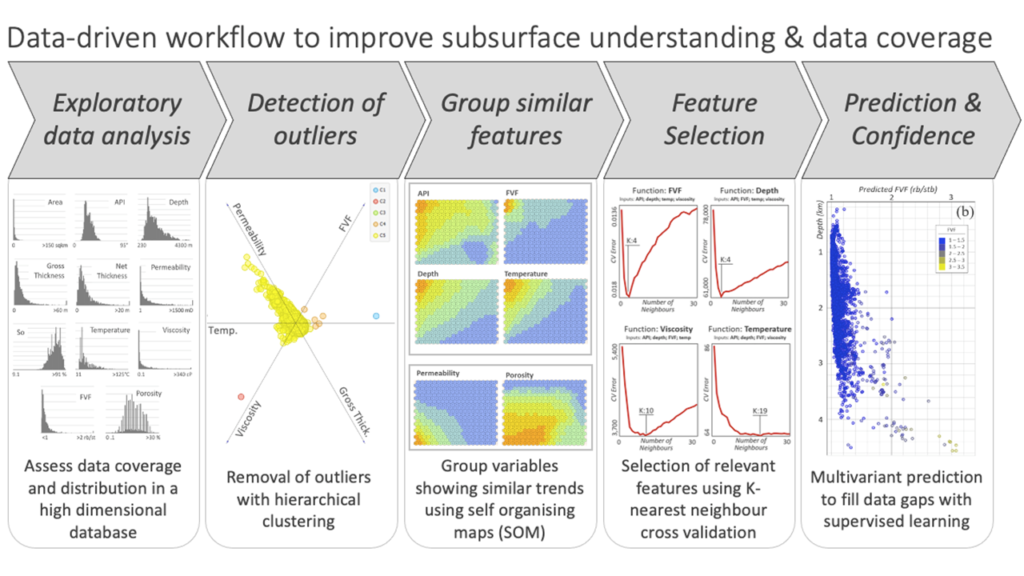
by Rachel E. Brackenridge, Vasily Demyanov, Oleg Vashutin, Ruslan Nigmatullin, Energies special issue on Machine Learning applications in reservoir modelling workflows, 2022, 15(3), 1070
Discover reservoir property relations from big data analytics of basin data to improve data coverage and prediction confidence. A general purpose bespoke machine learning workflow is designed to support business decision making processes in: (i) improve the data coverage by filling the gaps in large basin data bases of reservoir characteristics; (ii) evaluate the data adequacy; (iii) assess the uncertainty in reservoir characteristics to de-risk prospect evaluation. This work outcomes from the collaboration with Wood Mackenzie.
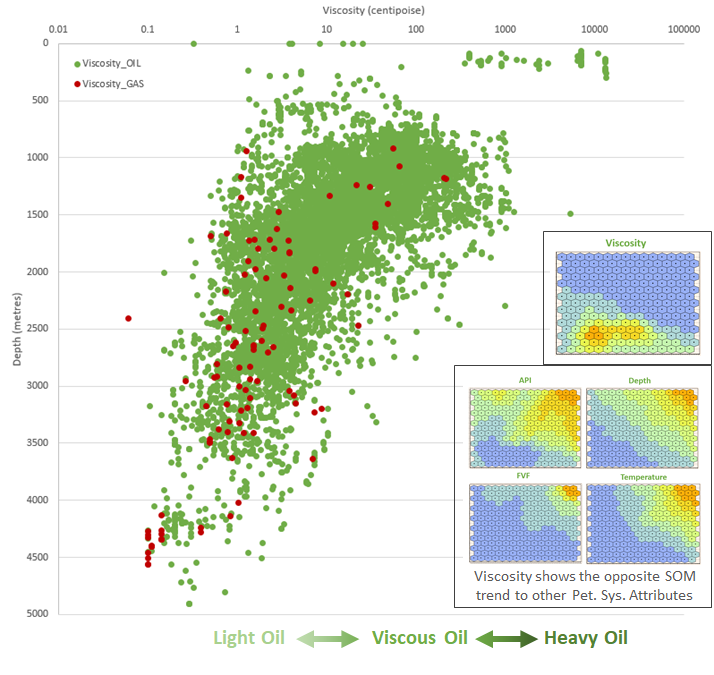
by Rachel E. Brackenridge, Vasily Demyanov, Oleg Vashutin, Ruslan Nigmatullin, Energies special issue on Machine Learning applications in reservoir modelling workflows, 2022, 15(3), 1070; https://doi.org/10.3390/en15031070