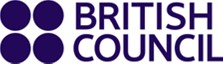
Warm-up Sessions
A series of warm-up sessions aim to set the context for the workshop discussion with keynote presentations and get the audience meet online and exchange views in breakout rooms.
The structure of each session includes:
- 10-min introduction breakout room
- 20 min keynote talk
- 15 min discussion breakout room
- Q&A and summaries from the room discussions and wrap up at the end
2 February, 2022, 10am GMT
Topological Data Analysis for Digital Rock Modeling and beyond
by Prof Evgeny Burnaev, Skolkovo Institute of Science and Technology, Moscow.
http://faculty.skoltech.ru/people/evgenyburnaev
Digital Rock Modeling requires fine analysis of core structure on different scales for reliable prediction of rock properties such as permeability.
Topological data analysis (TDA) is a recent field that emerged from various works in applied (algebraic) topology and computational geometry. TDA helps to perform multiscale analysis of the complex topological and geometric structures underlying data that are often represented as point clouds in Euclidean or more general metric spaces. In this talk, first, I will present ideas on how TDA can be used for permeability prediction. Then I will present some more advanced and general concepts of applying TDA to compare data manifolds that are useful for the analysis of generative models and artificial data generation.
Slide presentation:
Bio. Prof. Evgeny Burnaev obtained his MSc in Applied Physics and Mathematics from the Moscow Institute of Physics and Technology in 2006. After successfully defending his Ph.D. thesis in Foundations of Computer Science at the Institute for Information Transmission Problem RAS in 2008, Evgeny stayed with the Institute as head of IITP Data Analysis and Predictive Modeling Lab. Since 2007 Evgeny Burnaev carried out a number of successful industrial projects with Airbus, SAFT, IHI, and Sahara Force India Formula 1 team among others. Since 2016 Evgeny Burnaev works as Associate Professor of Skoltech. Currently, he is a director of a research center in AI, specializing in industrial analytics and development of machine learning methods. The main research directions are predictive modeling for ESG and optimization of technological processes, 3D computer vision, and data fusion. In 2017 Evgeny Burnaev was honored with the Moscow Government Prize for Young Scientists in the category “Transmission, Storage, Processing and Protection of Information” for his scientific contribution and leading the project “The development of methods for predictive analytics for processing industrial, biomedical and financial data.”
14 January, 2022, 10am GMT
Geoscience and data challenges in Subsurface Characterisation by Dr Dave McCarthy, British Geological Survey, Edinburgh
Geoscience and modelling the subsurface have been fundamental to Earth resource development for centuries, this has led to the shaping of society and our energy supply and material usage. Modern subsurface characterisation requires extensive geoscience skills and has generated huge volumes of large datasets. Similarly, the nature and type of geological data is changing rapidly. Large digital datasets and the availability of computing power have changed the way that geoscientists approach challenges.
As well as more traditional geoscience applications (hydrocarbons and mining), there are a growing number of challenges (Carbon Capture and Storage, Geological Disposal of Radioactive Waste, Energy Storage) that involve storage of fluids or waste in the subsurface rather than extraction.
Effective hydrocarbons exploration requires a blend of skills, including structural geology, sedimentology, geophysics and petrophysics to generate valid geological models of the subsurface from sparse datasets that can be interpreted in a number of non-unique ways. Carbon Capture and Storage requires a similar skillset, but rather than extracting and needing to ensure economic viability, there is emphasis on storage and ensuring CO2 does not escape. Similarly, the development of geothermal energy requires knowledge of rock and aquifer properties from even sparser datasets, with the added problems of needing to understand thermo-mechanical properties of rocks and fluid flow. Small deviations from predicted models may cause the development to be economically unviable. Infrastructure projects such as wind farms have similar problems when it comes to foundation design and emplacement.
An emerging field, the geological disposal of radioactive waste will be for many countries be their largest environmental protection projects, and will rightly require some of the most detailed and heavily scrutinised sub-surface analyses ever carried out in order to ensure safe and secure disposal for geological timescales.
These subsurface characterisation challenges are united in the large datasets generated and the number of models generated. There is a growing need for the skills, knowledge, and experience of geologists combined with data-driven science methods to facilitate sustainable growth and a net zero future. This talk will highlight some of the challenges and query whether we are asking the right questions?
15 December, 2021, 10am GMT
Data, data everywhere! Data driven challenges in Geoscience
by Prof Jennifer McKinley, Queen’s University, Belfast
7 December, 2021
Introduction by Vasily Demyanov and Q&A by Ivan Priezzhev
Design of a system to build a lithofacies model based on artificial intelligence elements
by Prof Ivan I. Priezzhev, Gubkin State Oil and Gas University, Moscow